
Installation instructions for Anaconda can be found here.Ī full list of the packages available as part of theĪnother advantage to installing Anaconda is that you don’t needĪdmin rights to install it. Rest of the SciPy stack without needing to installĪnything else, and without needing to wait for any software to be compiled.
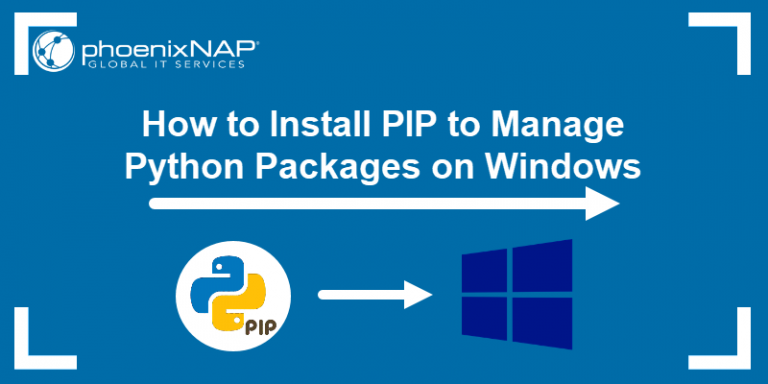
(Linux, macOS, Windows) Python distribution for data analytics andĪfter running the installer, the user will have access to pandas and the The simplest way to install not only pandas, but Python and the most popular Installing pandas and the rest of the NumPy and Installing pandas # Installing with Anaconda # PyPI, ActivePython, various Linux distributions, or aĭevelopment version are also provided.
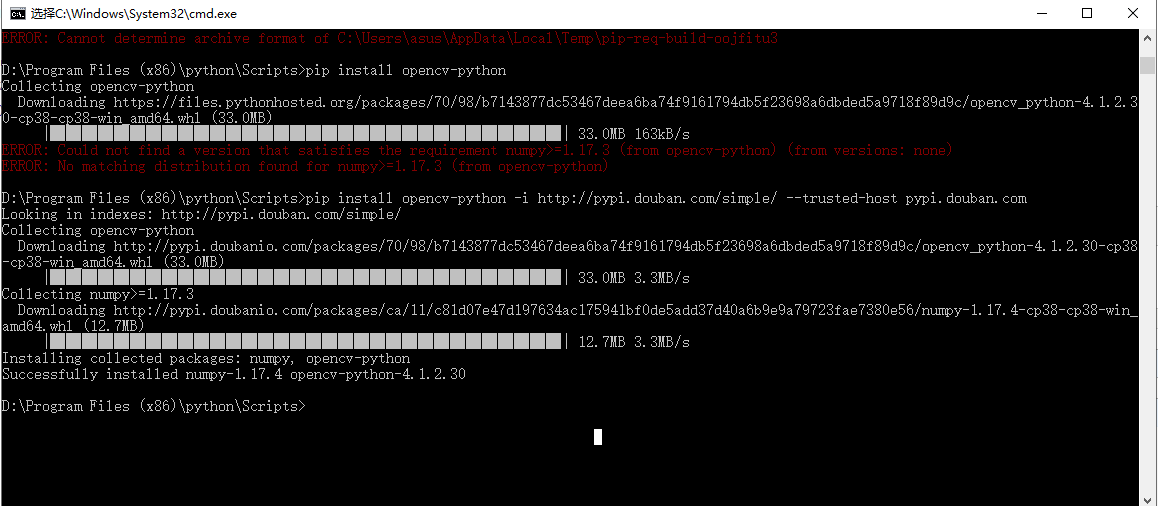
This is the recommended installation method for most users. Tensor learning, algebra and backends to seamlessly use NumPy, MXNet, PyTorch, TensorFlow or CuPy.The easiest way to install pandas is to install itĬross platform distribution for data analysis and scientific computing. Python backend system that decouples API from implementation unumpy provides a NumPy API. Manipulate JSON-like data with NumPy-like idioms. Multi-dimensional arrays with broadcasting and lazy computing for numerical analysis. NumPy-compatible sparse array library that integrates with Dask and SciPy's sparse linear algebra.ĭeep learning framework that accelerates the path from research prototyping to production deployment.Īn end-to-end platform for machine learning to easily build and deploy ML powered applications.ĭeep learning framework suited for flexible research prototyping and production.Ī cross-language development platform for columnar in-memory data and analytics. Labeled, indexed multi-dimensional arrays for advanced analytics and visualization NumPy-compatible array library for GPU-accelerated computing with Python.Ĭomposable transformations of NumPy programs: differentiate, vectorize, just-in-time compilation to GPU/TPU.

NumPy's API is the starting point when libraries are written to exploit innovative hardware, create specialized array types, or add capabilities beyond what NumPy provides.ĭistributed arrays and advanced parallelism for analytics, enabling performance at scale. With this power comes simplicity: a solution in NumPy is often clear and elegant. NumPy brings the computational power of languages like C and Fortran to Python, a language much easier to learn and use. Nearly every scientist working in Python draws on the power of NumPy.
